or otherwise known as:
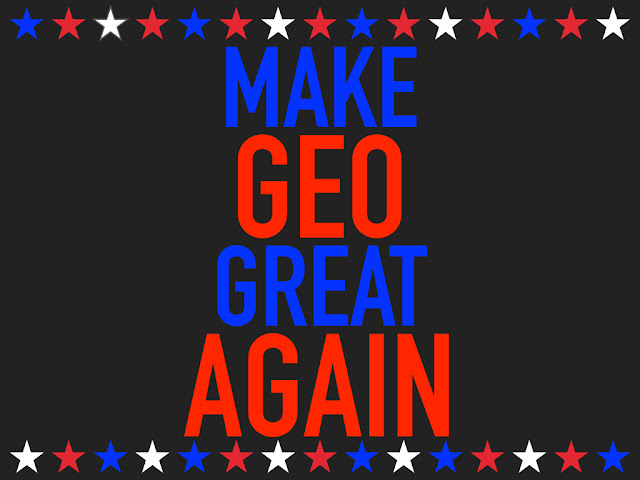
I started using docker when I first tried to build accumulo and geomesa on my MacBook, and like many projects involving compiling native binaries, it was a nightmare. I found an image of accumulo and geomesa on Docker Hub which I was able to use immediately. One of the barriers to adopting open source is the ability to run it on the system you have. Docker makes this possible with a minimum of effort.
Before diving into making geo great again, here are a few terms and definitions to establish a common vocabulary.
- Dockerfile: This tells the image builder (i.e Jenkins) what the image should look like.
- Image: The basis of a Docker container at rest. These artifacts are stored and managed in a registry. Once instantiated via a Docker run command a container is created.
- Container: The standard unit in which the application service resides. At run, the image is turned into a container.
- Docker Engine: Installed on physical, virtual or cloud hosts, this lightweight runtime is what pulls images, creates and runs containers.
- Registry: A service where Docker images are stored, managed and distributed.
Here's the tl;dr version. A Dockerfile is used to create an image, then an image running it's called a container, the Docker Engine is what is used to run an image, you can find images in the Docker Hub registry. Got it?
Here's the difference between a virtual machine and a container.
![]() |
You can install docker on Linux, OSX, Windows and cloud. If you just want to experiment, I encourage you to join the beta program.
So you've installed docker –
Let's do something useful, yet familiar; which is run gdal on docker.
docker run geodata/gdal
Since docker couldn't find the image locally, it downloaded the image and when it ran, it automatically ran gdalinfo to show that it was running. This is all very well and interesting but since there are binaries for gdal for most operating systems this isn't very exciting. So let's add a few more applications that will help with a popular geoprocessing task.
There has been quite a number of posts and recipes for creating natural color pan-sharpened images from Landsat. Most of these involve downloading and compiling several open source tools. For this example, we will take the the geodata/gdal image and add a few more tools and use a script to perform the image processing. An "enough to be dangerous" level of proficiency in git and linux is sufficient to do this.
First, fork and clone the the geodata/docker git repository from https://github.com/geo-data/gdal-docker/
Edit the Dockerfile to include dans-gdal-scripts and imagemagick:
# Install the application.
ADD . /usr/local/src/gdal-docker/
RUN apt-get update -y && \
apt-get install -y make && \
make -C /usr/local/src/gdal-docker install clean && \
apt-get purge -y make && \
apt-get install -y dans-gdal-scripts && \
apt-get install -y imagemagick
Do the git dance of add, commit, and push your updated Dockerfile. Or you can just fork and clone from the presentation repository.
Build the new image:
docker build -t spara/gdal:local git://github.com/spara/gdal-docker/
Next we will use an existing script to convert a Landsat 8 image to a pansharpened natural color jpeg suitable for framing. To use our new extra fancy docker image, we'll modify to script which can be found here. Note that I tweaked the settings to provide a brighter image.
Let's test out our new tool, for comparison we'll use a NASA tutorial and the data they used to make a natural color image. Here's the image from the tutorial:
and here's the image from our extra fancy docker gdal image.
I think that the image they used in their article is not the same as the link to their test image since that looks like a lot more snow cover and that this image is zoomed in in comparison to the NASA image.
With as little work as possible, we've ‘leveraged’ the work others by using and existing container and scripts, added more capabilities to a single image and created a single purpose tool that can deployed and reused anywhere. The image is availabe from Docker Hub.
docker pull spara/gdal_ef
We've built a tool that is reusable across any operating system and can be used as a 'Lego' brick when composing a data processing workflow. This is how we're going to make geo great again.
However, you don't even need to do this since there are already many geo tools available on Docker Hub
I'll end this with a quote